“The core advantage of data is that it tells you something about the world that you didn’t know before.”
Hilary Mason, data scientist and founder of Fast Forward Labs
Today, data is the need of the hour. No matter what sector a business operates in, accessing data is critical for steering it in the right direction and fostering success.
But is having this data enough to make informed business decisions?
The answer to that is a resounding ‘no’.
In our fast-paced world, where new information is produced every second, data becomes old in no time. The data you collected today might have some data points that are incomplete, scattered, or slightly incorrect the next time you check it.
So, how do we make sure your data always stays fresh and relevant?
The answer is data enrichment.
In this blog, we will see what data enrichment is & how it works. By the end of this read, you will have a solid understanding of why enriching your data stands as a pillar in smart business decision-making!
What is Data Enrichment?
Remember the last time you were typing in Google, and the search engine started guessing what you were going to write before you even finished?
That’s data enrichment in action!
It takes the little bit of data you give it and uses it to make a smart guess about what you want.
In layman’s words, data enrichment at its core is the process that turns raw data into actionable insights with freshness and accuracy.
As businesses start to grow, they start collecting a lot of information from basic contact details to customer buying preferences. But this data is much like a puzzle, often with several missing data points.
By enriching data you can fill in the gaps by pulling in additional, reliable information from various data sources, creating an up-to-date & accurate database.
Having access to this “richer” data can help businesses predict their growth in a certain defined timeframe. Further, it is easy to identify new opportunities businesses can tap into by identifying the market trends with this enriched data.
Often, times however data enrichment is confused with data cleaning.
While cleaning data is about removing what’s wrong or not needed, enriching it is about adding value, and making the data better and more insightful.
An Example of Data Enrichment
Let’s consider a company that desires to recruit the best talents in its domain. For the sake of this example let’s suppose the company has an arbitrary name “ABC Solutions” & is an AI solution company. They first identified other companies or related companies that may be using the talents ABC Solutions is looking for.
They note down the domains of these competing firms in their industry. Utilizing these domain addresses and feeding them into a data enrichment process, they manage to add layers of valuable information to their initial data (domain names), bringing in details about potential candidates.
See how enrichmentapi’s Employee Enrichment API can help you add multiple layers of information. (You only have to pass the domain names to this API & you get the employees list from a particular domain). Read the documentation for more details.
Read More: How Data Enrichment Can Help in Talent Recruitment
These details could be the current responsibilities, the projects potential hires have worked on, and the cities they are based in.
Now, instead of reaching out blindly, ABC Solutions can craft messages that not only highlight why the individual would be a perfect fit but also resonate with their personal and professional journey so far, thereby driving their recruitment strategy with enriched data, and significantly increasing the chances of securing interviews with industry-leading professionals.
In the above example, we illustrated how enriching with a single data point — the domain name of reputed companies — can be a transformative step in securing potential leads and fostering fruitful collaborations.
Now, imagine multiplying this strategy across various data points to create a web of interconnected and more insights.
6 Types of Data Enrichment
There are different types of data enrichment, some of which are:-
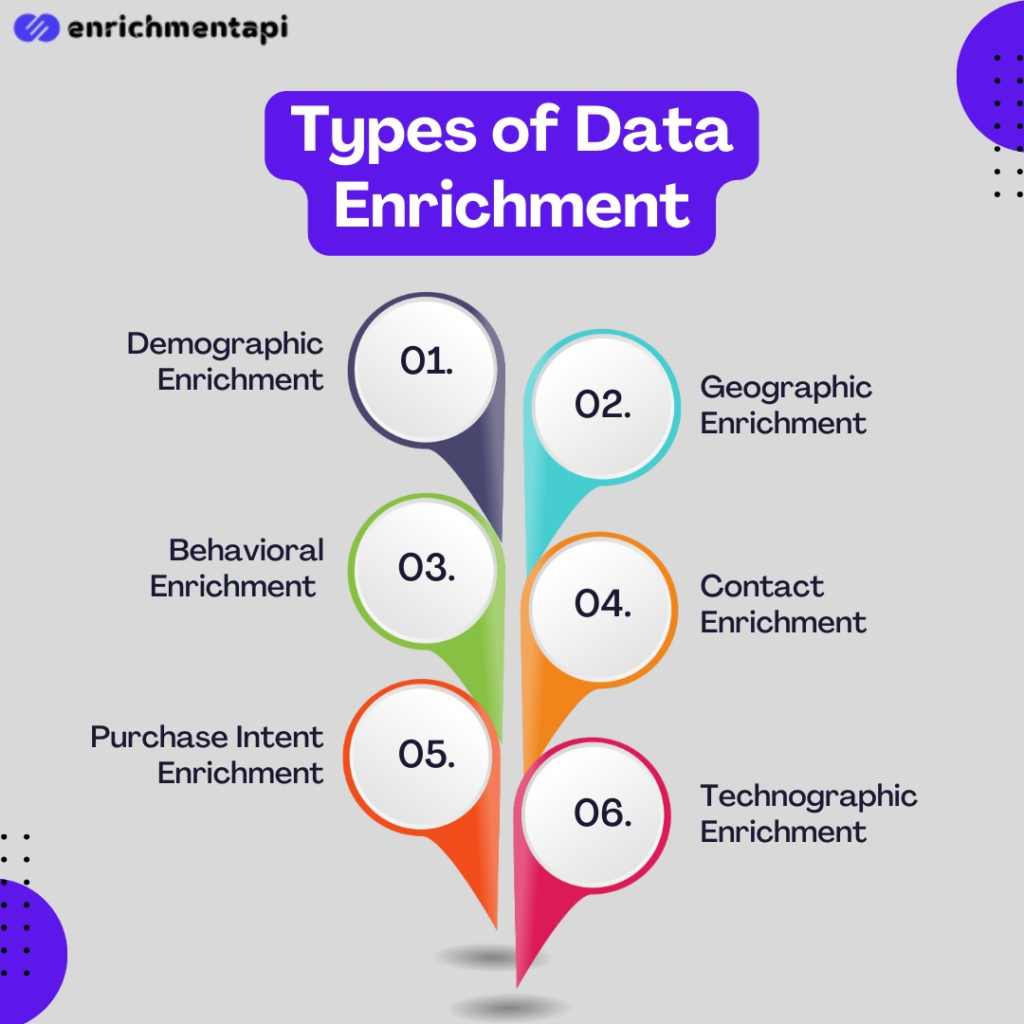
1. Demographic Enrichment
Demographic enrichment is enriching your database with comprehensive demographic information, such as age, income levels, and educational background, allowing for more segmented and personalized marketing strategies.
It helps in understanding the lifestyles and preferences of different customer groups, facilitating targeted and relatable content creation.
2. Geographic Enrichment
This type involves enhancing data sets with detailed geographical information like postal codes, city boundaries, or latitude and longitude details.
It aids businesses in understanding regional market dynamics and customer preferences, paving the way for region-specific marketing campaigns and strategic location planning for new outlets or offices.
3. Behavioral Enrichment
Behavioral enrichment adds patterns to your audience by adding data points reflecting their purchase history, time spent on shopping, browsing habits, and anything that is related to their online behavior.
It empowers businesses to craft personalized experiences and identify potential areas of interest for different customer segments, fostering a deeper connection and understanding of the customer journey.
4. Contact Enrichment
This type of enrichment augments your database by adding verified contact information such as business emails & job titles to the existing customer or lead data.
Further, it helps in maintaining an updated database, enabling efficient and effective communication strategies that resonate well with the recipients.
5. Purchase Intent Enrichment
By understanding and adding data regarding a potential customer’s readiness and willingness to make a purchase based on their shopping data and product view frequencies, businesses can create more focused and conversion-oriented marketing campaigns.
This enrichment type allows for the identification of warm leads, steering them closer to a buying decision through well-placed and timed strategies.
6. Technographic Enrichment
Technographic Enrichment involves understanding and adding data related to the company’s technological landscape. It encompasses details like the software and hardware used by a company, their tech-stack preferences, and digital tools adopted in their operations.
Technographic enrichment provides a competitive edge by helping to tailor offers and solutions that seamlessly integrate with a prospective company’s existing technology infrastructure.
7 Benefits of Enriching Data
Enriching data allows businesses to dig deeper into their customer perspective. There are several benefits of data enrichment, however, some common benefits are: –
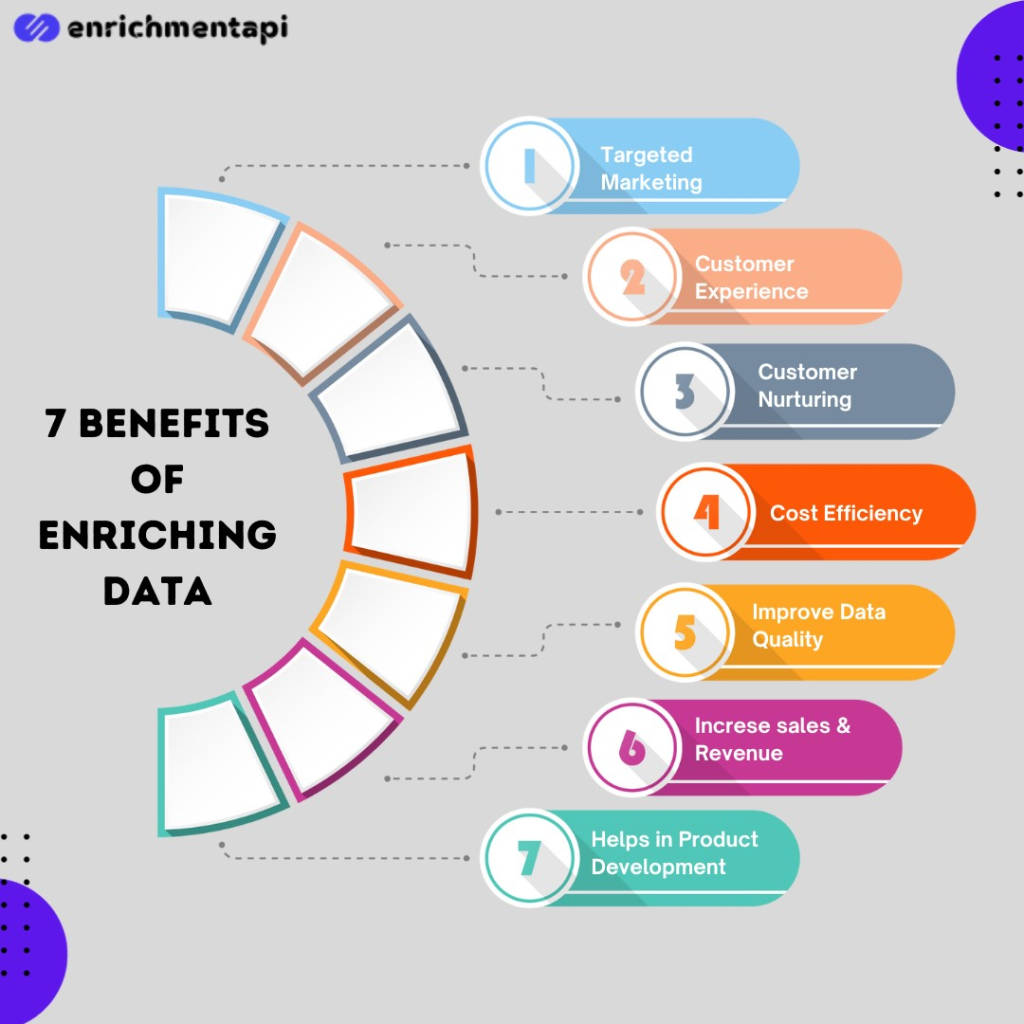
Enhanced Targeted Marketing
Data enrichment empowers businesses to identify specific and granular segments for targeted marketing, honing in on demographic details such as age ranges or income levels.
This facilitates more potent marketing strategies that resonate well with the target audience, thereby promising higher success rates in marketing campaigns.
Augmented Customer Experience
In a world where customers anticipate personalized experiences, enrichment can be handy with personalized content based on segmentation.
Helping businesses not just meet but exceed customer expectations, providing them with unmatched relevance and precision in the services and information offered.
Optimized Customer Nurturing
Identifying and nurturing potential customer segments becomes a streamlined process with data enrichment.
You can create value-driven content strategies that focus on invoking a genuine interest in the prospective customers, steering them closer to making a favorable purchasing decision.
Cost-Efficiency
Data enrichment significantly trims down the costs associated with managing voluminous yet irrelevant data. By enhancing internal data with valuable insights from external sources, organizations can wisely allocate their resources, focusing on fruitful endeavors.
Improved Data Quality
Adding a fresh dimension to data management, enrichment facilitates the elimination of redundant data, purging the system of duplications and inconsistencies.
This ensures a higher quality of data, which becomes a reliable foundation for business strategies, steering clear of pitfalls associated with misinformation.
Boosted Revenue Operations and Sales
Revenue operations see a notable enhancement with data enrichment laying a strong foundation for tech stacks. It fine-tunes lead scoring and routing processes, bringing contextual information into play to assign leads.
Furthermore, it ensures a clean and accurate contact list, optimizing sales efficiency and opening avenues for cross-sells and upsells.
Innovative Product Development
A less explored yet significant benefit of data enrichment is its role in product development. By digging deep insights into customer preferences and market trends, organizations can make their product development strategies, ensuring a product line that is not only innovative but also in sync with the market demands, hence promising a higher success rate post-launch.
Few Techniques used to enrich data
In this section, we will be looking at some of the techniques used to enrich data.
Appending Data
Appending data is one of the known data enrichment techniques, used in significantly enhancing the depth and reliability of existing datasets.
It involves the seamless integration of diverse data sources to form a more refined, accurate, and consistent dataset, surpassing the limitations of isolated data systems.
By collecting data from various systems such as CRM, financial, and marketing systems, businesses can construct a more detailed and holistic customer profile.
This multidimensional view allows for a richer understanding of customer behaviors, preferences, and needs, enabling more personalized and effective marketing strategies.
Another facet of appending data includes the infusion of data from third-party resources into your existing datasets.
Know More: How Right Data Sourcing Can Be a Game Changer for Your Business
This could range from demographic data, and geographical information like postcodes or ZIP codes, to more dynamic data such as weather or traffic conditions. Such external data, when merged accurately, provides additional layers of insight.
One more application of data appending lies in enriching location data. With a wealth of location-related data available globally, businesses can effortlessly integrate this information, offering more granular insights into geographical trends, customer movements, and potential market opportunities.
Apart from these, appending data can encompass various other dimensions including exchange rates, date/time hierarchies, and more.
Each addition not only fills the potential gaps in the existing data but also amplifies the scope for detailed analysis and informed decision-making.
Data Segmentation
Data segmentation is a critical method that divides a broad dataset into smaller, more manageable parts, allowing businesses to focus on specific aspects and draw actionable insights.
Here is a straightforward breakdown:
1. Defining Groups:
Data segmentation starts by dividing a data object (like a customer or product) into separate groups based on certain variables such as age, gender, or income. This grouping allows for a more precise classification and detailed description of the entity.
2. Using Segmentation:
After segmentation, businesses can utilize these distinct groups to gain a deeper understanding, leading to the creation of tailored strategies for different segments, and enhancing the effectiveness of marketing and customer service efforts.
3. Common Types of Segmentation:
A. Demographic Segmentation: This is based on variables like age, gender, and occupation.
B. Geographic Segmentation: This type is based on geographical information such as country, state, or city of residence.
C. Technographic Segmentation: This involves categorization based on technological preferences, like Apple vs. Android usage.
D. Psychographic Segmentation: This more subjective type is based on personal attitudes, values, and interests.
E. Behavioral Segmentation: This type is based on customer actions, such as spending habits or website usage patterns.
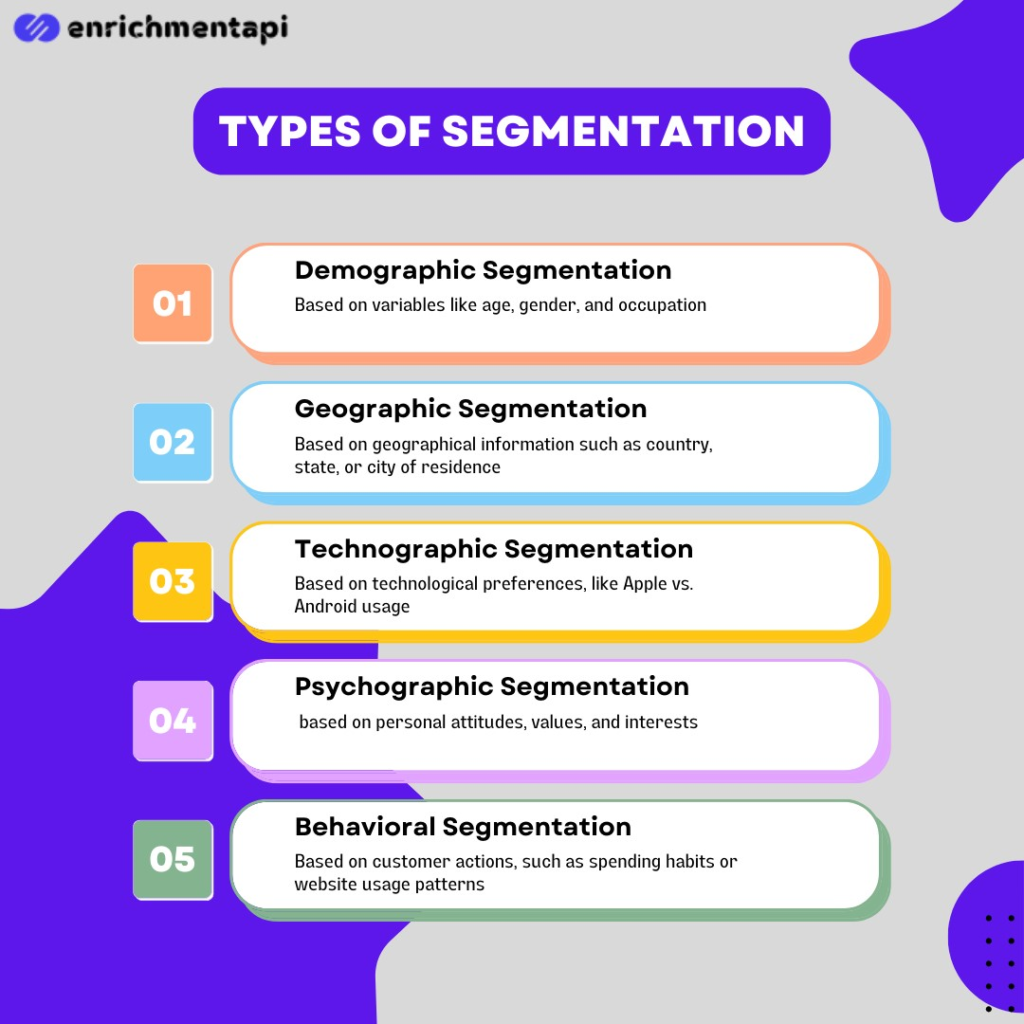
As per the data available to you, there can be more categories, these are the most commonly used and known categories.
4. Enhancing Personalization:
Beyond these, data segmentation can significantly boost personalization efforts. By understanding the different segments, businesses can craft more relevant and engaging messages and offers, leading to improved customer satisfaction and loyalty.
5. Facilitating Better Analysis:
Additionally, segmentation helps in better data analysis. It allows companies to analyze specific data segments for trends, patterns, and insights that may be obscured in a larger dataset. It’s a step towards making more informed and strategic business decisions.
Derived Attributes
Derived attributes offer a new layer of insight from existing datasets. Let’s understand derived attributes and see how they play a substantial role in enhancing data analysis and reporting capabilities.
Derived attributes are additional fields in a dataset that don’t have storage in the original dataset but are formulated from one or more existing fields. They provide more refined, actionable insights without the need for external data sourcing.
For example, a customer’s age is not directly stored in data. However, it can be efficiently derived from the customer’s date of birth, allowing businesses to effortlessly categorize their customer base by age brackets for targeted marketing or product personalization.
Derived attributes pave the way for quicker, more efficient data analysis. They eliminate the recurring need for manual calculations, substantially reducing the time it takes to perform new analyses and ensuring timely decision-making.
These attributes aid in maintaining consistency and relevancy in the used analysis measures.
Their standardized nature guarantees uniformity in the metrics used for data analysis, ensuring the reliability of the insights gained.
Derived attributes can span various types, including counter fields, date-time conversions, and time-between calculations. These derived metrics bolster the analysis capabilities, offering multiple insights from a single dataset.
Their inclusion in the data enrichment process significantly improves data reporting. The added depth and context to the existing data facilitate a more robust and comprehensive data analysis process.
Imputation
Imputation is a straightforward but essential process in data enrichment. It’s about filling in the blanks!!
When data is missing or inconsistent in certain fields, imputation helps by substituting these gaps with estimated values. This step is crucial to avoid any distortion in data analysis.
For instance, consider a situation where an order’s value is missing. Instead of leaving it blank or counting it as zero, we can estimate the value.
How?
By looking at the customer’s past purchases or analyzing the value of similar bundles of goods. This method helps in keeping the analysis accurate and reliable.
In addition, imputation aids in maintaining the integrity of the dataset. It ensures that the dataset remains complete and consistent, which is vital for making informed business decisions.
Entity Extraction
Entity extraction is a technique utilized to clean valuable structured information from data that is unstructured or semi-structured. This method helps in pinpointing and identifying specific entities within a large volume of data.
Examples of these entities include people, places, organizations, and certain numerical expressions like dates, time, and monetary amounts.
Suppose you have a large set of customer feedback about various restaurants. This feedback is rich with information but is in a form that’s hard to analyze.
With entity extraction, you can easily pull out the names and locations of the restaurants mentioned in the feedback.
This way, specific and valuable information is efficiently extracted from a bulk of text, making it easier for analysis and review.
Adding to the examples, if there’s a mention of the date and time when a customer visited a restaurant, entity extraction can pull out this information too. This can help businesses analyze trends and make more informed decisions.
By identifying such precise and critical information from a heap of data, businesses can enhance their operations, customer service, and various other aspects.
This method is not just limited to certain types of information. It’s versatile and can be adapted to various scenarios and needs, making it a significant technique in data enrichment.
Data Categorization
Data categorization is crucial for making sense of unstructured data, and converting it into a structured and more understandable form.
Here’s a concise explanation, ensuring clarity and ease of understanding for all readers:
It is the act of sorting and labeling data to make it organized and easier to analyze. It helps turn messy information into a clear, structured form. This process usually falls into two main parts:
1. Sentiment Analysis:
- What is it? It’s a method to find out the mood or feeling of a piece of text.
- Why do it? To know if customers are happy, upset, or neutral about services or products.
- Example: Reading a review to see if a customer liked a restaurant or not.
2. Topication:
- What is it? It’s finding out what a piece of text is mainly talking about.
- Why do it? Organize information based on its main subject.
- Example: Figuring out if a news article is about sports, politics, or something else.
This process makes sure no valuable information gets lost in the shuffle of lots of data. It helps businesses know what customers are saying and thinking, making it easier to meet their needs and keep them happy.
Conclusion
Data enrichment can help in different industries in various ways. The key is to get the fresh and relevant data possible every time.
The seamless integration of enriched data translates to a richer customer experience, streamlined operations, and an unwavering forward momentum in the business journey.
Comments are closed.